Benjamin Riley: AI is Another Ed Tech Promise Destined to Fail
Deans for Impact founder urges educators to ‘calm down’ and resist the ‘overhype and magical thinking’ that often accompany innovation.
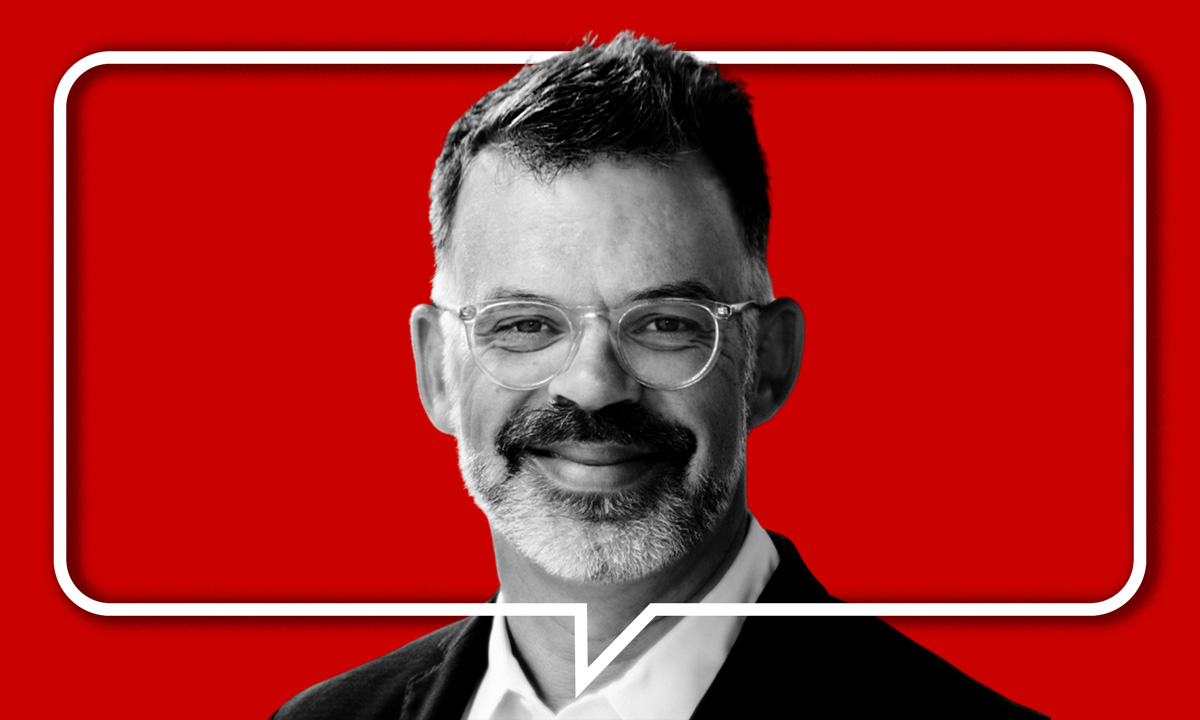
Get stories like these delivered straight to your inbox. Sign up for The 74 Newsletter
For more than a decade, Benjamin Riley has been at the forefront of efforts to get educators to think more deeply about how we learn.
As the founder of Deans for Impact in 2015, he enlisted university education school deans to incorporate findings from cognitive science into teacher preparation. Before that, he spent five years as policy director of the NewSchools Venture Fund, which underwrites new models of schooling. In his new endeavor, Cognitive Resonance, which he calls “a think-and-do tank,” he’s pushing to help people think not only about how we learn, but how generative artificial intelligence (AI) works — and why they’re different.
His Substack newsletter and Twitter feed regularly poke holes in high-flying claims about the power of AI-powered tutors — he recently offered choice words for Khan Academy founder Sal Khan’s YouTube demonstration of Open AI’s new GPT4o tool, saying it was “deployed in the most favorable educational environment we can possibly imagine,” leaving open the possibility that it might not perform so well in the real world.
In April, Riley ruffled feathers in the startup world with an essay in the journal Education Next that took Khan Academy and other AI-related companies to task for essentially using students as guinea pigs.
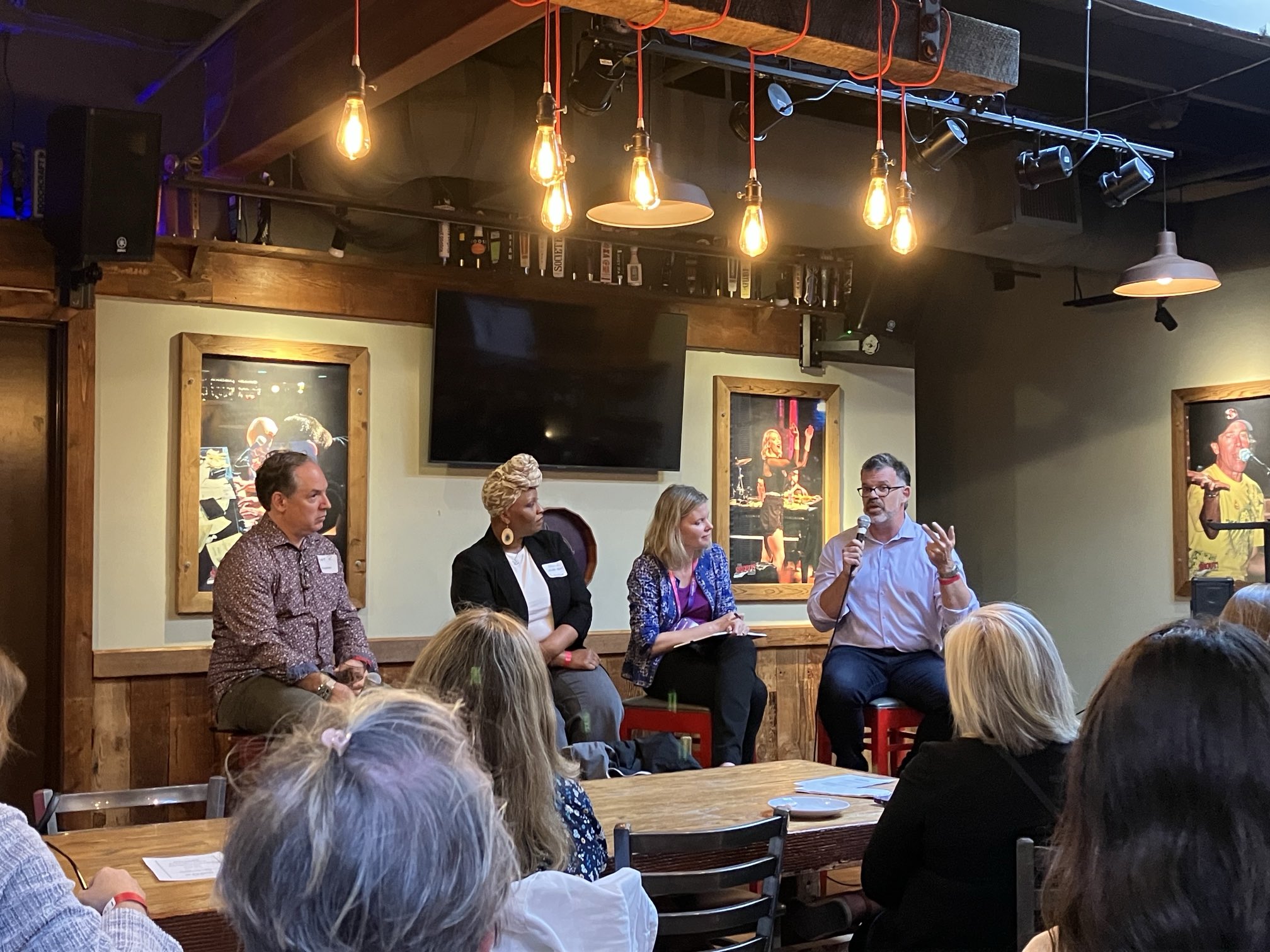
In the essay, he recounted asking Khanmigo to help him simplify an algebraic equation. Riley-as-student got close to solving it, but the AI actually questioned him about his steps, eventually asking him to rethink even basic math, such as the fact that 2 + 2.5 = 4.5.
Such an exchange isn’t just unhelpful to students, he wrote, it’s “counterproductive to learning,” with the potential to send students down an error-filled path of miscalculation, misunderstanding and wasted effort.
The interview has been edited for length and clarity.
The 74: We’re often so excited about the possibilities of ed tech in education that we just totally forget what science says about how we learn. I wonder if you have any thoughts on that.
Benjamin Riley: I have many. Part of my frustration is that we are seemingly living in a moment where we’re simultaneously recognizing in other dimensions where technology can be harmful, or at least not beneficial, to learning, while at the same time expressing unbridled enthusiasm for a new technology and believing that it finally will be the cure-all, the silver bullet that finally delivers on the vision of radically transforming our education system. And yeah, it’s frustrating. Ten years ago, for example, when everybody was excited about personalization, there were folks, myself included, raising their hand and saying, “Nope, this doesn’t align with what we know about how we think and learn. It also doesn’t align with the science of how we collectively learn, and the role of education institutions as a method of culturally transmitting knowledge.” All of those personalized learning dreams were dying out. And many of the prominent, incredibly well-funded personalized learning efforts either went completely belly-up, like AltSchool, or have withered on the vine, like some of the public schools now named Gradient.
Now AI has revived all of those dreams again. And it’s frustrating, because even if it were true that personalization were the solution, no one 10 years ago, five years ago, was saying, “But what we need are intelligent chatbot tutors to make it real.” So what you’re seeing is sort of a commitment to a vision. Whatever technology comes along, we’re going to shove into that vision and say that this is going to deliver it. I think for the same reasons it failed before, it will fail again.
You’re a big fan of the University of Virginia cognitive scientist Daniel Willingham, who has done a lot to popularize the science of how we learn.
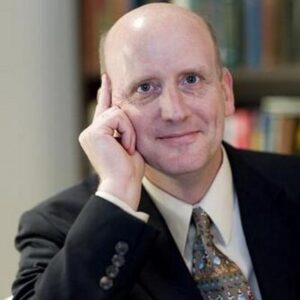
He’s wonderful at creating pithy phrases that get to the heart of the matter. One of the counterintuitive phrases he has that is really powerful and important is that our minds in some sense “are not built to think,” which feels really wrong and weird, because isn’t that what minds do? It’s all they do, right? But what he means is that the process of effortful thinking is taxing in the same way that working out at the gym is taxing. One of the major challenges of education is: How do you wrap around that with students, who, like all of us, are going to try to essentially avoid doing effortful thinking for sustained periods? Over and over again, technologists just assume away that problem.
In the case of something like large language models, or LLMs, how do they approach this problem of effortful thinking? Do they just ignore it altogether?
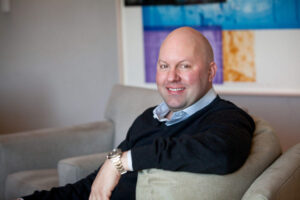
It’s an interesting question. I’m almost not sure how to answer it, because there is no thinking happening on the part of an LLM. A large language model takes the prompts and the text that you give it and tries to come up with something that is responsive and useful in relation to that text. And what’s interesting is that certain people — I’m thinking of Mark Andreessen most prominently — have talked about how amazing this is conceptually from an education perspective, because with LLMs you will have this infinitely patient teacher. But that’s actually not what you want from a teacher. You want, in some sense, an impatient teacher who’s going to push your thinking, who’s going to try to understand what you’re bringing to any task or educational experience, lift up the strengths that you have, and then work on building your knowledge in areas where you don’t yet have it. I don’t think LLMs are capable of doing any of that.
As you say, there’s no real thinking going on. It’s just a prediction machine. There’s an interaction, I guess, but it’s an illusion. Is that the word you would use?
Yes. It’s the illusion of a conversation.
In your Education Next essay, you quote the cognitive scientist Gary Marcus, who says LLMs are “frequently wrong, but never in doubt.” It feels to me like that is extremely dangerous in something young people interact with.
Yes! Absolutely. This is where it’s really important to distinguish between the now and the real and the present versus the hypothetical imagined future. There’s just no question that right now, this “hallucination problem” is endemic. And because LLMs are not thinking, they generate text that is factually inaccurate all the time. Even some of the people who are trying to push it out into the world acknowledge this, but then they’ll just put this little asterisk: “And that’s why an educator must always double-check.” Well, who has the time? I mean, what utility is this? And then people will say, “Well yes, but surely it’s going to get better in the future.” To which I say, Maybe, let’s wait and see. Maybe we should wait until we’ve arrived at that point before we push this out.
Do we know how often LLMs are making mistakes?
I can say just from my own personal usage of Khanmigo that it happens a lot, for reasons that are frankly predictable once you understand how the technology works. How often is it happening with seventh-grade students who are just learning this idea for the first time? We just don’t know. [In response to a query about errors, Khan Academy sent links to two blog posts on its site, one of which noted that Khanmigo “occasionally makes mistakes, which we expected.” It also pointed, among other things, that Khanmigo now uses a calculator to solve numerical problems instead of using AI’s predictive capabilities.]
One of the things you say in the EdNext piece is that you just “sound like a Luddite” as opposed to actually being one. The Luddites saw the danger in automation and were trying to push against it. Is it the same, in a way, as what you’re doing?
Thank you for asking that question because I feel my naturally contrarian ways risk painting me into a corner I’m really not in. Because in some sense, generative AI and large language models are incredible — they really are. It is a remarkable achievement that they are able to produce fluent and coherent narratives in response to just about any combination of words that you might choose to throw at them. So I am not a Luddite who thinks that we need to burn this all down.
“You want an impatient teacher who’s going to push your thinking, try to understand what you’re bringing to any task or educational experience, lift up the strengths that you have, and then work on building your knowledge in areas where you don’t yet have it. I don’t think LLMs are capable of doing any of that.”
There are methods and ways, both within education and in society more broadly, in which this tool could be incredibly useful for certain purposes. Already, it’s proving incredibly stimulating in thinking about and understanding how humans think and learn, and how that is similar and different from what they do. If we could just avoid the ridiculous overhype and magical thinking that seems to accompany the introduction of any new technology and calm down and investigate before pushing it out into our education institutions, then I think we’d be a lot better off. There really is a middle ground here. That’s where I’m trying to situate myself.
Maybe this is a third rail that we shouldn’t be touching, but I was reading about Thomas Edison and his ideas on education. He had a great quote about movies, which he thought would revolutionize classrooms. He said, “The motion picture will endure as long as poor people exist.” It made me think: One of the underlying themes of ed tech is this idea of bringing technology to the people. Do you see a latent class divide here? Rich kids will get an actual personal tutor, but everybody else will get an LLM?
My worry runs differently than that. Again, back to the Willingham quote: “Our minds are not built to think.” Here’s the harsh reality that could indeed be a third rail, but it needs to be acknowledged if we’re going to make meaningful progress: If we fail in building knowledge in our students, thinking gets harder and harder, which is why school gets harder and harder, and why over time you start to see students who find school really miserable. Some of them drop out. Some of them stop trying very hard. These folks — the data is overwhelming on this — typically end up having lives that are shorter, with less economic means, more dire health outcomes. All of this is both correlated and interrelated causation.
“If we could just avoid the ridiculous overhype and magical thinking that seems to accompany the introduction of any new technology and investigate before pushing it out into our education institutions, then I think we’d be a lot better off.”
But here’s the thing: For those students in particular, a device that alleviates the cognitive burden of schooling will be appealing. I’m really worried that this now-widely available technology will be something they turn to, particularly around the incredibly cognitively challenging task of writing — and that they will continue to look to this as a way of automating their own cognition. No one really needs to worry about the children of privilege. They are the success stories academically and, quite frankly, many of them enjoy learning and thinking and will avoid wanting to use this as a way of outsourcing their own thinking. But it could just make the existing divide a lot wider than it is today — much wider.
How is education research responding to AI?
The real challenge is that the pace of technology, particularly the pace of technological developments in the generative AI world, is so fast that traditional research methods are not going to be able to keep up. It’s not that there won’t be studies — I’m sure there are already some underway, and there’s tiny, emerging studies that I have seen here and there. But we just don’t have the capabilities as a research enterprise to be doing things the traditional way. A really important question that needs to be grappled with, as a matter of policy, potentially as a matter of philanthropy and just as a matter of society, is: So, what then? Do we just do it and hope for the best? Because that may be what ends up happening.
As we’ve seen with social media and smartphones in schools, there can be real impacts that you don’t realize until five, 10 years down the road. Then you go back and say, “Well, I wish we’d been thinking about that in advance rather than just rolling the dice and seeing where it came up.” We don’t do that in other realms of life. We don’t let people just come up with medicines that they think will cure certain diseases and then just say, “Well, we’ll see. We’ll introduce it into broader society and let’s figure it out.” I’m not necessarily saying that we need the equivalent per se, but something that would give us better insight and real-time information to help us figure out the overall positives and not-so-positives seems to me a real challenge that is underappreciated at the moment.
Get stories like these delivered straight to your inbox. Sign up for The 74 Newsletter